OVERVIEW
The CORSMAL Challenge: Multi-modal Fusion and Learning for Robotics | |
Apostolos Modas Pascal Frossard Andrea Cavallaro Ricardo Sanchez-Matilla Alessio Xompero | EPFL, CH EPFL, CH QMUL, UK QMUL, UK QMUL, UK |
QA4Camera: Quality assessment for Smartphone Cameras | |
Wenhan Zhu Xiongkuo Min Guangtao Zhai | SJTU, China SJTU, China SJTU, China |
Embedded Deep Learning Object Detection Model Compression Competition for Traffic in Asian Countries | |
Ted Kuo, Jenq-Neng Hwang, Jiun-In Guo, Chia-Chi Tsai | NCTU, Taiwan NCTU, Taiwan NCTU, Taiwan NCTU, Taiwan |
Encoding in the Dark | |
Nantheera Anantrasirichai Paul Hill Angeliki Katsenou Fan Zhang | Univ Bristol, UK Univ Bristol, UK Univ Bristol, UK Univ Bristol, UK |
Densely-sampled Light Field Reconstruction | |
Prof. Atanas Gotchev | Tampere UT, Fi |
CHALLENGE 1
Title: The CORSMAL Challenge: Multi-modal Fusion and Learning for Robotics
Summary:
A major challenge for human-robot cooperation in household chores is enabling robots to predict the properties of containers with different fillings. Examples of containers are cups, glasses, mugs, bottles and food boxes, whose varying physical properties such as material, stiffness, texture, transparency, and shape must be inferred on-the-fly prior to a pick-up or a handover. The CORSMAL challenge focuses on the estimation of the pose, dimensions and mass of containers.
We will distribute a multi-modal dataset with visual-audio-inertial recordings of people interacting with containers, for example while pouring a liquid in a glass or moving a food box. The dataset is recorded with four cameras (one on a robotic arm, one worn by the person and two third-person views) and a microphone array. Each camera provides RGB, depth and stereo infrared images, which are temporally synchronized and spatially aligned. The body-worn camera is equipped with an inertial measurement unit, from which we provide the data as well.
The challenge focuses on the estimation of the pose, dimensions and mass of containers. Participants will determine the physical properties of a container while it is manipulated by a human. Containers vary in their physical properties (shape, material, texture, transparency and deformability). Containers and fillings are not known to the robot, and the only prior information available is a set of object categories (glasses, cups, food boxes) and a set of filling types (water, sugar, rice).
The challenge includes three scenarios:
Scenario 1: A container is on the table, in front of the robot. A person pours filling into the container or shakes an already filled food box, and then hands it over to the robot.
Scenario 2: A container is held by a person, in front of the robot. The person pours the filling into the container or shakes an already filled food box, and then hands it over to the robot.
Scenario 3: A container is held by a person, in front of the robot. The person pours filling into the container or shakes an already filled food box, and then walks around for a few seconds holding the container. Finally, the person hands the container over to the robot.
Each scenario is recorded with two different backgrounds and under two different lighting conditions.
Challenge webpage: http://corsmal.eecs.qmul.ac.uk/ICME2020_Challenge.html
Organisers: Apostolos Modas, Pascal Frossard (EPFL, CH), Andrea Cavallaro, Ricardo Sanchez-Matilla, Alessio Xompero (QMUL, UK)
CHALLENGE 2
Title: QA4Camera: Quality assessment for Smartphone Cameras
Summary:
Smartphones have been one of the most popular digital devices in the past decades, with more than 300 million sold every quarter world-wide. Most of the smartphone vendors, such as Apple, Huawei, Samsung, launch new flagship smartphones every year. People use smartphone cameras to shoot selfie photos, film scenery or events, and record videos of family and friends. The specifications of smartphone camera and the quality of taken pictures are major criteria for consumer to select and buy smartphones. Many smartphone manufacturers also introduce and advertise their smartphones by introducing the strengths and advantages of their smartphone cameras. However, how to evaluate the quality of smartphone cameras and the pictures taken remains a problem for both smartphone manufacturers and consumers. Currently in the market, there are several teams and companies who evaluate the quality of smartphone cameras and announce the ranking and scores of the quality of smartphone cameras, and the scores of smartphone cameras are subjectively graded by several photographers and experts from different aspects, such as exposure, color, noise and texture. However, subjective assessment is not easy to reproduce, and it is not easy to deploy in practical image processing systems.
In the last two decades, objective image quality assessment (IQA) has been widely researched, and a large number of objective IQA algorithms have been designed to automatically and accurately estimate the quality of images. However, most objective IQA methods are designed to assess the overall perceived quality of the image degraded by various simulated distortions, which rarely exist in pictures taken by the modern smartphone cameras. Thus these methods are not suitable for the task of smartphone camera quality assessment, while objective evaluation methods specifically designed for the purpose of smartphone camera quality assessment are relatively rare.
The purpose of this Grand Challenge is to drive efforts of image quality assessment towards smartphone camera quality assessment. With this Grand challenge, it is expected to develop objective smartphone camera quality assessment models from four different aspects, including exposure, color, noise and texture, by using the datasets released by the organizers. The goal is to provide reference quality rankings or scores for smartphone cameras and to both smartphone manufacturers and consumers.
Participants are asked to submit four computational models to calculate the rankings of smartphone camera from four aspects: exposure, color, noise and texture.
Datasets and further information: https://qa4camera.github.io/
Organisers: Wenhan Zhu, Xiongkuo Min, Guangtao Zhai (SJTU, China)
CHALLENGE 3
Title: Embedded Deep Learning Object Detection Model Compression Competition for Traffic in Asian Countries
Summary:
Object detection in the computer vision area has been extensively studied and making tremendous progress in recent years using deep learning methods. However, due to the heavy computation required in most deep learning-based algorithms, it is hard to run these models on embedded systems, which have limited computing capabilities. In addition, the existing open datasets for object detection applied in ADAS applications usually include pedestrian, vehicles, cyclists, and motorcycle riders in western countries, which is not quite similar to the crowded Asian countries with lots of motorcycle riders speeding on city roads, such that the object detection models trained by using the existing open datasets cannot be directly applied in detecting moving objects in Asian countries.
In this competition, we encourage the participants to design object detection models that can be applied in the competition’s traffic with lots of fast speeding motorcycles running on city roads along with vehicles and pedestrians. The developed models not only can fit for embedded systems but also can achieve high accuracy at the same time.
This competition is divided into two stages: qualification and final competition.
- Qualification competition: all participants submit their answers online. A score is calculated. The top 15 teams would be qualified to enter the final round of the competition
- Final competition: the final score will be validated and evaluated by the organizing team over NVIDIA Jetson TX2 for the final score
The goal is to design a lightweight deep learning model suitable for constrained embedded system design to deal with traffic in Asian countries. We focus on detection accuracy, model size, computational complexity and performance optimization on NVIDIA Jetson TX2 based on a predefined metric.
Given the test image dataset, participants are asked to detect objects belonging to the following four classes {pedestrian, vehicle, scooter, bicycle} in each image, including class and bounding box.
Datasets and further information: https://pairlabs.ai/icme2020-grand-challenge/
Organisers: Ted Kuo, Jenq-Neng Hwang, Jiun-In Guo, Chia-Chi Tsai (NCTU, Taiwan)
CHALLENGE 4
Title: Encoding in the Dark
Summary:
Low light scenes often come with acquisition noise, which not only disturbs the viewers, but it also makes video compression harder. These type of videos are often encountered in cinema as a result of artistic perspective or the nature of a scene. Other examples include shots of wildlife (e.g. mobula rays at night in Blue Planet II), concerts and shows, surveillance camera footage and more. Inspired by all above, we are proposing a challenge on encoding low-light captured videos. This challenge intends to identify technology that improves the perceptual quality of compressed low-light videos beyond the current state of the art performance of the most recent coding standards, such as HEVC, AV1, VVC etc. Moreover, this will offer a good opportunity for both experts in the fields of video coding and image enhancement to address this problem. A series of subjective tests will be part of the evaluation, the results of which can be used in a study of the tradeoff between artistic direction and the viewers’page preferences, such as mystery movies and some investigation scenes in the film. Participants will be requested to deliver bitstreams with pre-defined maximum target rates for a given set of sequences, a short report describing their contribution and a software executable for running the proposed methodology and then can reconstruct the decoded videos by the given timeline. Participants are also encouraged to submit a paper for publication in the proceedings, and the best performers shall be prepared to present a summary of the underlying technology during the ICME session. The organisers will cross-validate and perform subjective tests to rank participant contributions.
Challenge webpage: https://bit.ly/2CdEbqb
Organisers: Nantheera Anantrasirichai, Paul Hill, Angeliki Katsenou, Fan Zhang (University of Bristol, UK)
CHALLENGE 5
Title: Densely-sampled Light Field Reconstruction
Summary:
A Densely-Sampled Light Field (DSLF) is a discrete representation of the 4D approximation of the plenoptic function parameterized by two parallel planes (camera plane and image plane), where multi-perspective camera views are arranged in such a way that the disparities between adjacent views are less than one pixel. DSLF allows generating any desired light ray along the parallax axis by simple local interpolation. DSLF capture settings in terms of physical camera locations depends on the minimal scene depth and the camera sensor resolution. The number of cameras can be high especially for capturing wide field of view content.
DSLF is an attractive representation of scene visual content, particularly for applications which require ray interpolation and view synthesis. The list of such applications includes refocusing, novel view generation for free-viewpoint video (FVV), super-multiview and light field displays and holographic stereography. Direct DSLF capture of real-world scenes requires a very high number of densely located cameras, which is not practical. This motivates the problem of DSLF reconstruction from a given sparse set of camera images utilizing the properties of the scene objects and the underlying plenoptic function.
The goal of this challenge is two-fold:
First, to generate high-quality and meaningful DSLF datasets for further experiments;
Second, to quantify the state of the art in the area of light field reconstruction and processing in order to provide instructive results about the practical LF capture settings in terms of number of cameras and their relative locations. This will be furthermore helpful in applications, aiming at:
- Predicting intermediate views from neighboring views in LF compression
- Generating high-quality content for super-multiview and LF displays
- Providing FVV functionality
- Converting LF (I.e. ray optics based) representation into holographic (I.e. wave optics based) representations for the needs of digital hologram generation
Proponents are asked to develop and implement algorithms for DSLF reconstruction from decimated-parallax imagery in three categories:
- Cat1: close camera views along parallax axis resulting in adjacent images with narrow disparity (e.g. in the range of 8 pixels)
- Cat2: moderately-distant cameras along parallax axis, resulting in adjacent images with moderate disparities (in the range of 15-16 pixels)
- Cat3: distant cameras, resulting in adjacent images with wide disparity (in the range of 30-32 pixels)
Algorithms in each category will be evaluated separately, thus resulting in three sub-challenges. Proponents are invited to submit solutions to one or more categories.
Datasets and further information: http://civit.fi/icme-2020-grand-challenge-on-densely-sampled-light-field-reconstruction
Organisers: Prof. Atanas Gotchev (Tampere UT, Finland)
GRAND CHALLENGE CHAIRS
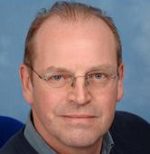
University of Bristol, UK
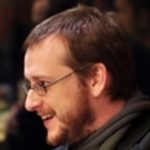
University of Nantes, France